Book Downloads Hub Reads Ebooks Online eBook Librarys Digital Books Store Download Book Pdfs Bookworm Downloads Free Books Downloads Epub Book Collection Pdf Book Vault Read and Download Books Open Source Book Library Best Book Downloads Wendy Smith S Daly Bob Tarte Graham Parkes Rick Walton Mary Meinking Jesse Stone Michael Leytonstone
Do you want to contribute by writing guest posts on this blog?
Please contact us and send us a resume of previous articles that you have written.
Revolutionizing Energy Demand Forecasting with Hybrid Intelligent Technologies: A Complete Guide

In today's ever-evolving world, the demand for energy has soared unprecedentedly. Industries, households, and commercial entities heavily rely on a steady supply of electricity to function efficiently. Energy consumption patterns and predicting future demand play a vital role in balancing supply and demand. This is where hybrid intelligent technologies step in, revolutionizing energy demand forecasting in profound ways.
Understanding Energy Demand Forecasting:
Energy demand forecasting involves estimating future electricity consumption to aid in resource planning, grid management, and policymaking for a sustainable future. Traditional forecasting methods heavily relied on statistical models based on historical data. While these models served their purpose to some extent, the need for more accurate, real-time predictions gave rise to hybrid intelligent technologies.
What are Hybrid Intelligent Technologies?
Hybrid intelligent technologies combine the strengths of multiple intelligent techniques, such as artificial intelligence (AI),machine learning (ML),and expert systems (ES),to enhance the accuracy and reliability of energy demand forecasting. These technologies leverage big data analytics, pattern recognition, and advanced algorithms to analyze a wide array of variables influencing energy consumption.
5 out of 5
Language | : | English |
File size | : | 39660 KB |
Text-to-Speech | : | Enabled |
Enhanced typesetting | : | Enabled |
Print length | : | 328 pages |
Screen Reader | : | Supported |
X-Ray for textbooks | : | Enabled |
Benefits of Hybrid Intelligent Technologies:
1. Increased Accuracy: Unlike traditional forecasting methods, hybrid intelligent technologies have the ability to process and analyze vast amounts of data in real-time. This allows for more accurate predictions, minimizing errors and providing a clear understanding of future energy demand patterns.
2. Adaptability: Hybrid intelligent technologies can adapt to changing variables and market dynamics. This enables them to address unexpected events like weather changes, natural disasters, and shifts in consumer behavior, ensuring a more reliable forecasting process.
3. Scalability: With the increasing demand for energy, traditional forecasting methods struggle to handle complexities and scale accordingly. Hybrid intelligent technologies have the capability to handle large datasets and complex models, making them scalable solutions for energy demand forecasting.
4. Cost-Efficiency: Accurate energy demand forecasting helps optimize resource allocation and grid management. By utilizing hybrid intelligent technologies, utilities can reduce wastage, minimize reliance on expensive backup power sources, and optimize energy distribution, resulting in significant cost savings.
Applications of Hybrid Intelligent Technologies in Energy Demand Forecasting:
1. Load Forecasting: Hybrid intelligent technologies are employed in load forecasting to predict electricity consumption patterns. By considering various factors such as historical data, time series analysis, weather conditions, and socio-economic variables, these technologies provide granular load forecasts that aid power grid planning and optimization.
2. Renewable Energy Integration: The variable nature of renewable energy sources like solar and wind makes integration into the power grid challenging. Hybrid intelligent technologies analyze historical weather patterns, solar radiation data, wind speed, and other relevant variables to optimize renewable energy integration and ensure a stable and reliable power supply.
3. Risk Assessment: Energy demand forecasting also plays a crucial role in risk assessment for utilities. By accurately predicting future demand, utilities can ensure they have enough backup power sources, assess potential revenue losses, and strategize their operations accordingly. Hybrid intelligent technologies with their adaptive capabilities offer valuable insights for risk assessment.
The Future of Energy Demand Forecasting:
The realm of hybrid intelligent technologies in energy demand forecasting is ever-evolving. As technology advances, more sophisticated algorithms, machine learning models, and AI-driven solutions will emerge, further enhancing accuracy and adaptability. Additionally, the integration of Internet of Things (IoT) devices and smart meters will provide real-time data, allowing for more precise predictions.
It is clear that hybrid intelligent technologies have immense potential to revolutionize energy demand forecasting. The ability to accurately predict electricity consumption patterns will enable utilities and policymakers to make informed decisions, optimize energy resources, and promote a sustainable energy future.
:
Hybrid intelligent technologies are reshaping the landscape of energy demand forecasting. By harnessing the power of AI, ML, and expert systems, these technologies are revolutionizing accuracy, adaptability, scalability, and cost-efficiency. Their applications extend to load forecasting, renewable energy integration, and risk assessment, enabling utilities to optimize operations and ensure a sustainable energy supply.
As we move towards a smarter and greener future, hybrid intelligent technologies will continue to evolve and play a crucial role in meeting the world's growing energy needs. Embracing these technologies is paramount for utilities and policymakers to effectively manage resources and foster a sustainable energy ecosystem.
5 out of 5
Language | : | English |
File size | : | 39660 KB |
Text-to-Speech | : | Enabled |
Enhanced typesetting | : | Enabled |
Print length | : | 328 pages |
Screen Reader | : | Supported |
X-Ray for textbooks | : | Enabled |
This book is written for researchers and postgraduates who are interested in developing high-accurate energy demand forecasting models that outperform traditional models by hybridizing intelligent technologies.
It covers meta-heuristic algorithms, chaotic mapping mechanism, quantum computing mechanism, recurrent mechanisms, phase space reconstruction, and recurrence plot theory.
The book clearly illustrates how these intelligent technologies could be hybridized with those traditional forecasting models. This book provides many figures to deonstrate how these hybrid intelligent technologies are being applied to exceed the limitations of existing models.
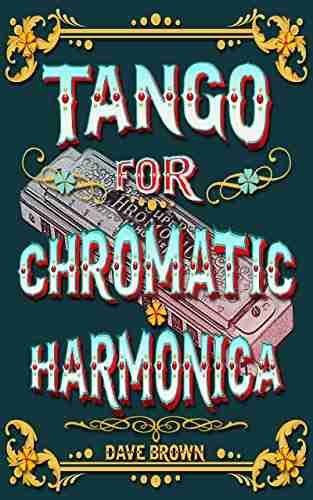

Tango For Chromatic Harmonica Dave Brown: Unleashing the...
The hauntingly beautiful sound of the...
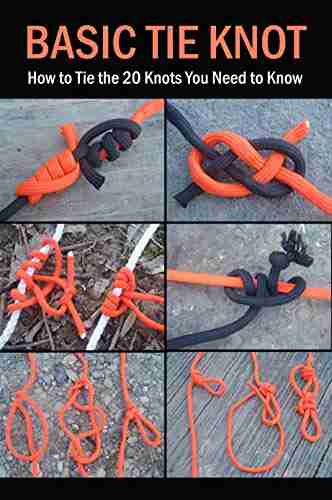

How To Tie The 20 Knots You Need To Know
Knot-tying is an essential...
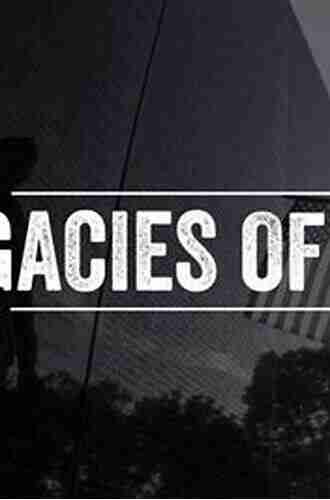

The Politics Experiences and Legacies of War in the US,...
War has always had a profound impact...
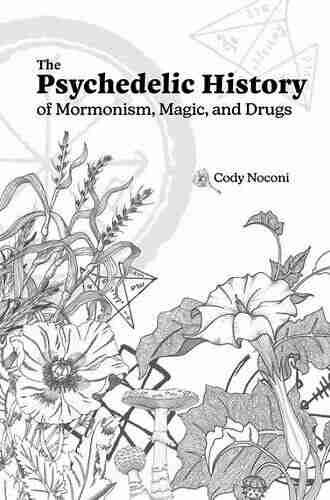

The Psychedelic History Of Mormonism Magic And Drugs
Throughout history, the connections between...
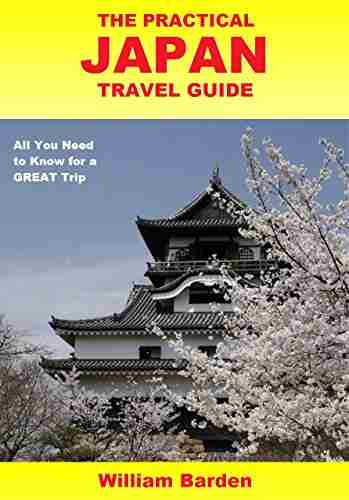

The Practical Japan Travel Guide: All You Need To Know...
Japan, known for its unique...
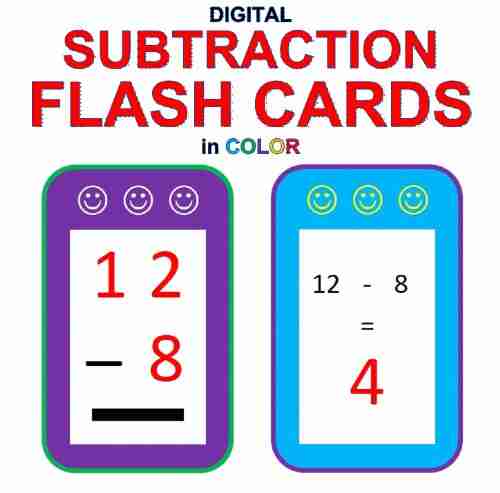

Digital Subtraction Flash Cards in Color: Shuffled Twice...
Mathematics is an essential...
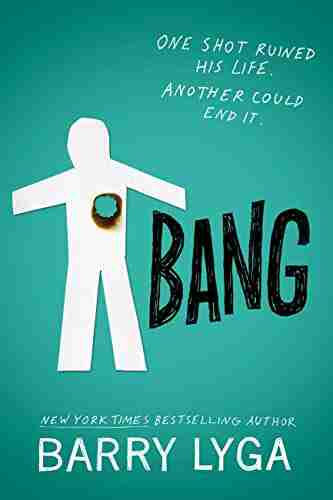

Unveiling the Enigma: Explore the Fascinating World of...
Hello, dear readers! Today, we have a...
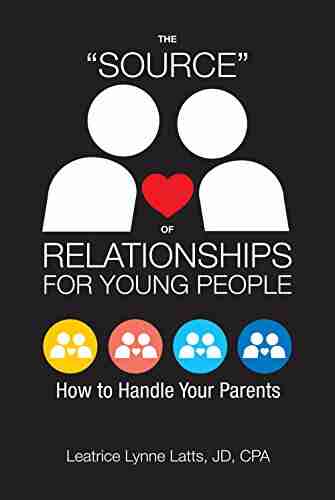

How To Handle Your Parents - A Comprehensive Guide
Are you having trouble dealing with your...
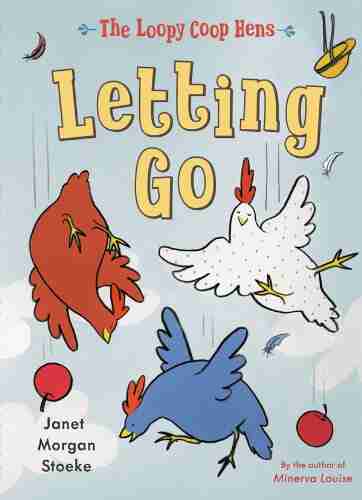

The Loopy Coop Hens Letting Go: A Tale of Friendship and...
Once upon a time, in a peaceful...
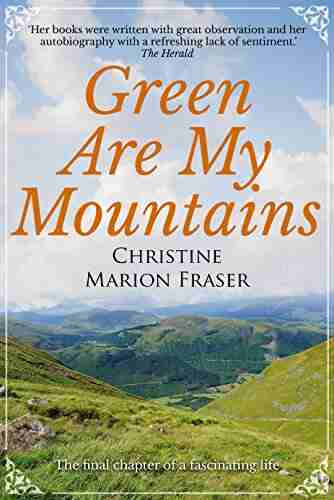

Green Are My Mountains: An Autobiography That Will Leave...
Are you ready to embark on an...
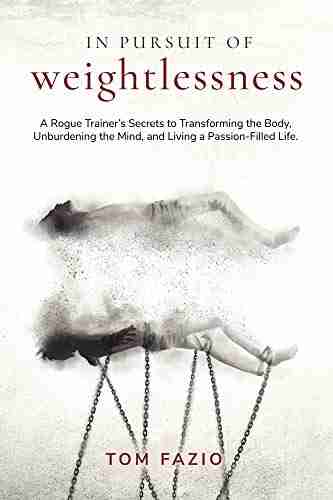

Rogue Trainer Secrets To Transforming The Body...
In this fast-paced...
Light bulbAdvertise smarter! Our strategic ad space ensures maximum exposure. Reserve your spot today!
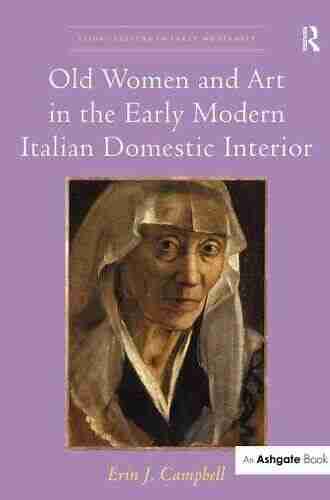

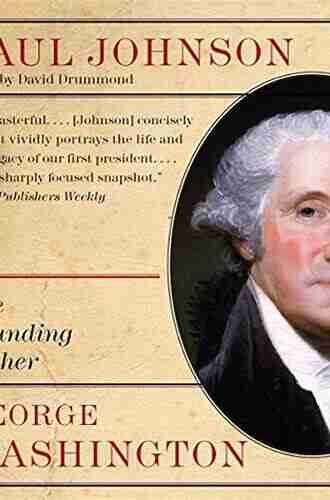

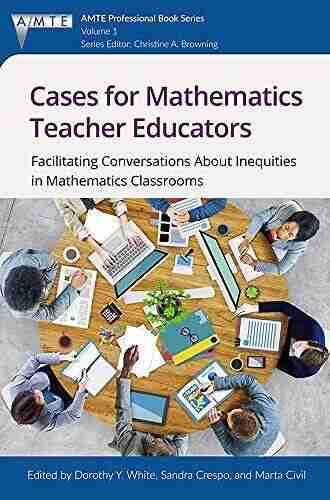

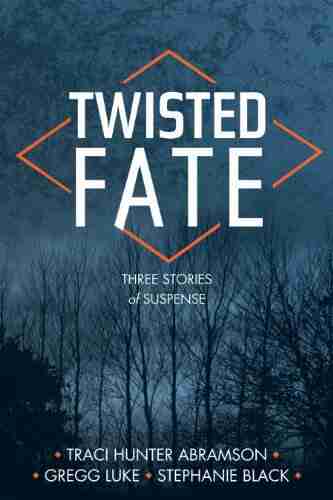

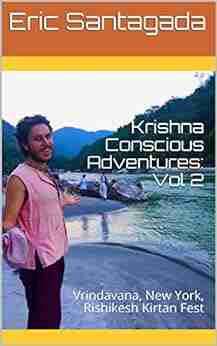

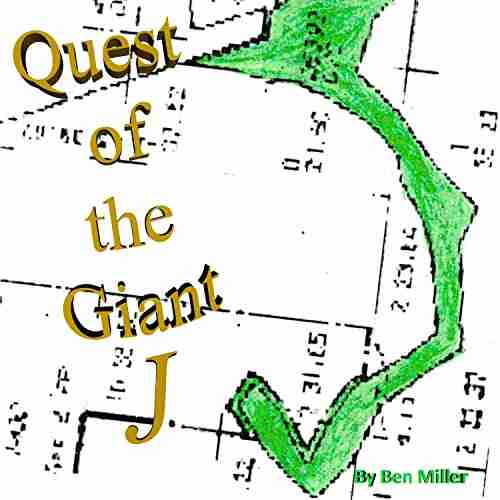

- Harvey HughesFollow ·17.2k
- Charles ReedFollow ·10.1k
- Garrett BellFollow ·4.6k
- Andy ColeFollow ·15.8k
- Samuel Taylor ColeridgeFollow ·11k
- Samuel WardFollow ·19.9k
- Tyler NelsonFollow ·9.3k
- Floyd PowellFollow ·3.4k