Book Downloads Hub Reads Ebooks Online eBook Librarys Digital Books Store Download Book Pdfs Bookworm Downloads Free Books Downloads Epub Book Collection Pdf Book Vault Read and Download Books Open Source Book Library Best Book Downloads Alison Light Suzanne Lahl Adrienne Maria Vrettos Katie Heaney George Acquaah Hemang Doshi A S Syla Holly Lloyd
Do you want to contribute by writing guest posts on this blog?
Please contact us and send us a resume of previous articles that you have written.
Unlocking the Potential: Reinforcement Learning Of Bimanual Robot Skills

Robotics and automation have revolutionized various industries, enabling enhanced efficiency, accuracy, and productivity. One area of particular interest is the field of Reinforcement Learning (RL) applied to bimanual robot skills. This cutting-edge technique holds great promise in advancing automation to new heights, as it empowers robots to perform complex tasks with two hands simultaneously, much like humans.
In recent years, researchers have made significant strides in developing RL algorithms and applying them to bimanual robot systems. The goal is to create robots that can learn from their interactions with the environment, improve their performance over time, and adapt to unforeseen challenges. By fusing the principles of machine learning and robotics, these advancements have the potential to transform various industries, from manufacturing to healthcare.
Understanding Reinforcement Learning (RL)
Reinforcement Learning is a critical branch of machine learning where agents interact with an environment to learn optimal actions in pursuit of achieving specific goals. Unlike other learning methods that rely on pre-defined instructions, RL enables robots to learn from trial and error, gradually refining their skills through positive and negative rewards.
4 out of 5
Language | : | English |
File size | : | 43813 KB |
Text-to-Speech | : | Enabled |
Enhanced typesetting | : | Enabled |
Print length | : | 273 pages |
Screen Reader | : | Supported |
RL employs a reward system, where robots receive feedback based on their actions. Positive rewards are given for desirable actions, whereas negative rewards are assigned for incorrect or undesired behaviors. Consequently, the robot gradually adjusts its actions to maximize rewards and minimize penalties, leading to improved performance and skill mastery.
The Power of Bimanual Robot Skills
Bimanual robot systems refer to robots that possess two arms or manipulators, similar to human counterparts. This capability opens up vast possibilities in various industries where tasks requiring dexterity and precision can be accomplished more efficiently. Some common scenarios where bimanual robot skills are highly valuable include assembly line tasks, surgery, household chores, and even tasks in extreme environments.
By leveraging RL techniques, these bimanual robots can learn to execute multi-step tasks that involve intricate coordination between both hands. For example, in manufacturing settings, bimanual robots can assemble complex products much faster and with higher precision compared to their single-arm counterparts.
Relevance of Reinforcement Learning Of Bimanual Robot Skills
One notable work in this field is the book titled Reinforcement Learning Of Bimanual Robot Skills Springer Tracts In Advanced Robotics. Edited by Arash Ajoudani and Alessandro De Luca, this comprehensive resource discusses the latest advancements and research contributions in RL techniques for bimanual robot systems.
The book highlights the challenges and opportunities in developing RL algorithms capable of leveraging bimanual capabilities. With contributions from leading experts in the field, it offers a deep dive into the various RL algorithms, architectures, and applications specifically tailored for bimanual robot skills.
Furthermore, the authors emphasize the long-term benefits of RL in bimanual robot systems, including increased productivity, improved human-robot collaboration, and enhanced task automation. The incorporation of RL techniques empowers robots to learn from human demonstrations and optimize their actions, paving the way for intelligent robots capable of executing complex tasks independently or collaboratively with humans.
The Future of Automation: Advancements and Challenges
As reinforcement learning continues to evolve, researchers aim to overcome several challenges to unlock the full potential of bimanual robot skills. Some key areas of exploration within the field include:
1. Handling Complex and Dynamic Environments
Bimanual robot systems must navigate uncertain, dynamic environments where objects can move, change, or have ambiguous properties. Reinforcement learning algorithms need to adapt to such environments and perform reliably despite these challenges.
2. Fostering Human-Robot Collaboration
Efficient collaboration between humans and robots is crucial, especially in tasks that require both parties to work together seamlessly. Bimanual robot systems equipped with RL techniques should adapt to human demonstrations, preferences, and ensure safe interactions throughout the collaborative process.
3. Efficient Transfer Learning Across Tasks
Transfer learning plays a vital role in RL algorithms, allowing robots to apply previously learned skills to new tasks or environments. Developing efficient methods to transfer knowledge between different tasks or between different robot systems is a focus area for researchers.
Addressing these challenges and further refining RL techniques for bimanual robot systems will accelerate the adoption of advanced automation across industries.
The possibilities and potential of reinforcement learning applied to bimanual robot systems are immense. The book Reinforcement Learning Of Bimanual Robot Skills Springer Tracts In Advanced Robotics provides a comprehensive overview of the latest advancements and research in this exciting field.
With the continued integration of RL techniques, bimanual robot systems will transform the automation landscape, empowering robots to perform intricate tasks with unrivaled precision and efficiency. The collaboration between researchers from multiple disciplines will shape the future of advanced automation, making our lives easier, safer, and more productive.
4 out of 5
Language | : | English |
File size | : | 43813 KB |
Text-to-Speech | : | Enabled |
Enhanced typesetting | : | Enabled |
Print length | : | 273 pages |
Screen Reader | : | Supported |
This book tackles all the stages and mechanisms involved in the learning of manipulation tasks by bimanual robots in unstructured settings, as it can be the task of folding clothes.
The first part describes how to build an integrated system, capable of properly handling the kinematics and dynamics of the robot along the learning process. It proposes practical enhancements to closed-loop inverse kinematics for redundant robots, a procedure to position the two arms to maximize workspace manipulability, and a dynamic model together with a disturbance observer to achieve compliant control and safe robot behavior.
In the second part, methods for robot motion learning based on movement primitives and direct policy search algorithms are presented. To improve sampling efficiency and accelerate learning without deteriorating solution quality, techniques for dimensionality reduction, for exploiting low-performing samples, and for contextualization and adaptability to changing situations are proposed.
In sum, the reader will find in this comprehensive exposition the relevant knowledge in different areas required to build a complete framework for model-free, compliant, coordinated robot motion learning.
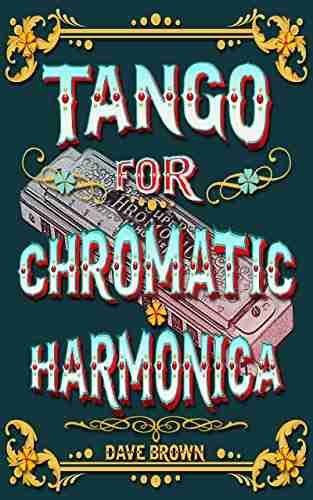

Tango For Chromatic Harmonica Dave Brown: Unleashing the...
The hauntingly beautiful sound of the...
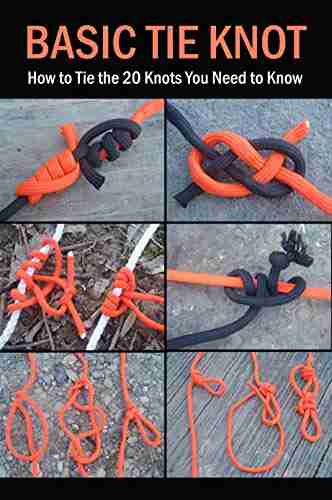

How To Tie The 20 Knots You Need To Know
Knot-tying is an essential...
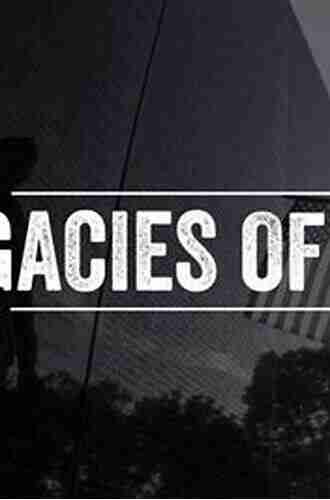

The Politics Experiences and Legacies of War in the US,...
War has always had a profound impact...
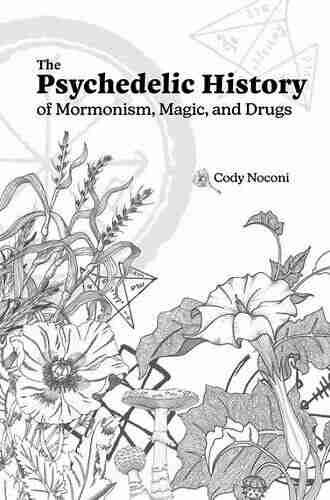

The Psychedelic History Of Mormonism Magic And Drugs
Throughout history, the connections between...
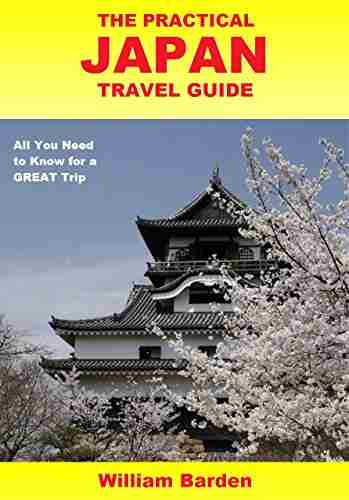

The Practical Japan Travel Guide: All You Need To Know...
Japan, known for its unique...
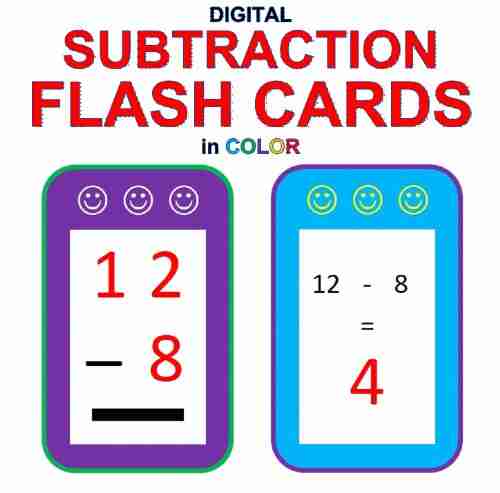

Digital Subtraction Flash Cards in Color: Shuffled Twice...
Mathematics is an essential...
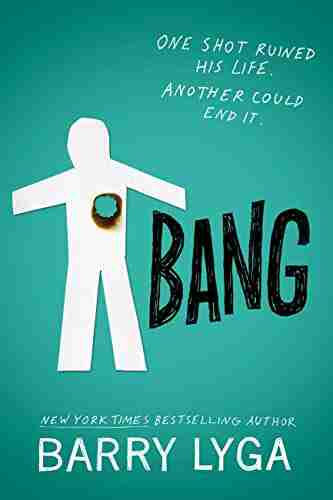

Unveiling the Enigma: Explore the Fascinating World of...
Hello, dear readers! Today, we have a...
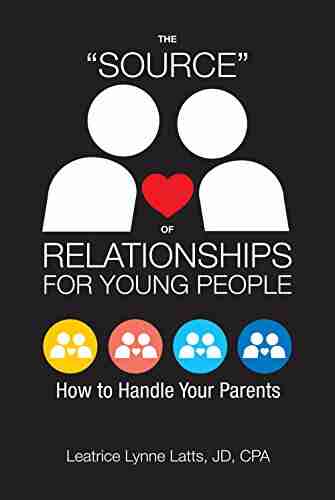

How To Handle Your Parents - A Comprehensive Guide
Are you having trouble dealing with your...
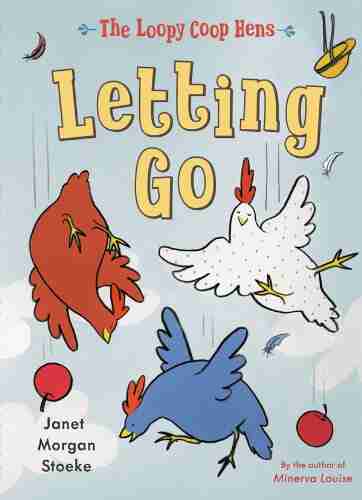

The Loopy Coop Hens Letting Go: A Tale of Friendship and...
Once upon a time, in a peaceful...
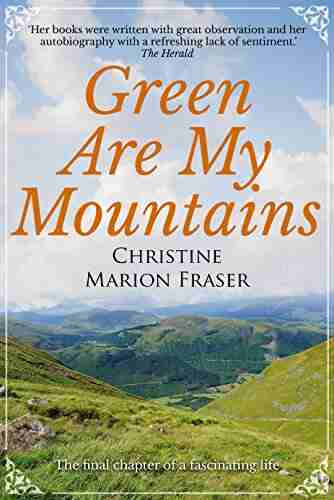

Green Are My Mountains: An Autobiography That Will Leave...
Are you ready to embark on an...
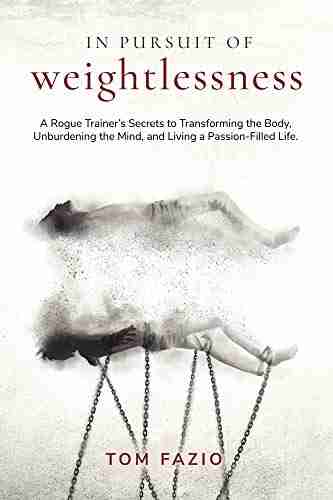

Rogue Trainer Secrets To Transforming The Body...
In this fast-paced...
Light bulbAdvertise smarter! Our strategic ad space ensures maximum exposure. Reserve your spot today!
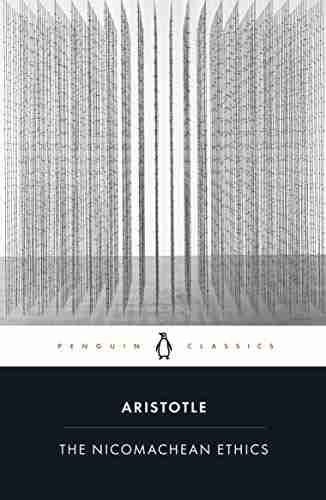

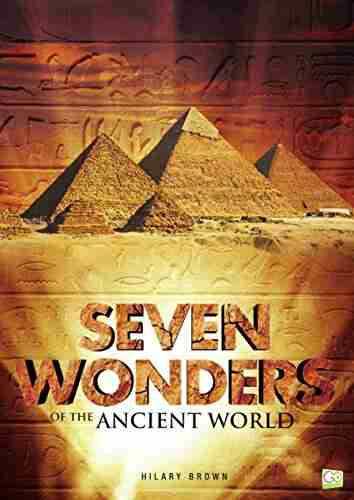

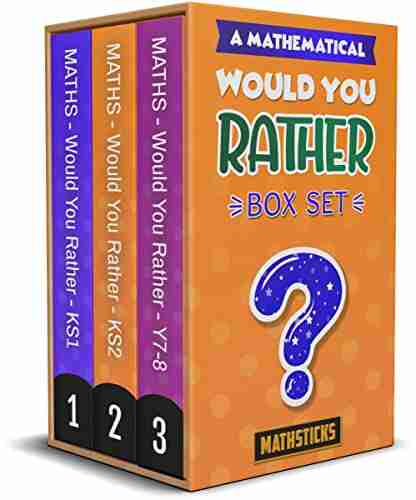

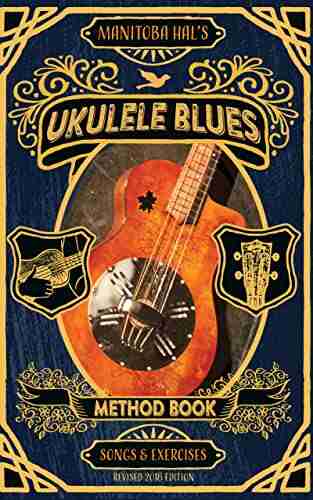

- Thomas PynchonFollow ·4.8k
- Elliott CarterFollow ·7.4k
- George BellFollow ·8.1k
- Eric HayesFollow ·14.4k
- Esteban CoxFollow ·19.6k
- Frank MitchellFollow ·18.6k
- E.M. ForsterFollow ·12.9k
- Jayson PowellFollow ·11.1k