Book Downloads Hub Reads Ebooks Online eBook Librarys Digital Books Store Download Book Pdfs Bookworm Downloads Free Books Downloads Epub Book Collection Pdf Book Vault Read and Download Books Open Source Book Library Best Book Downloads A G Henley Jaya P N Bishwal Austen Hartke Wenceslas S Jardetzky Aalia Zealous Andra Damron Nicholas Wise Meghan Power
Do you want to contribute by writing guest posts on this blog?
Please contact us and send us a resume of previous articles that you have written.
Parameter Estimation In Stochastic Differential Equations Lecture Notes In: A Comprehensive Guide

Stochastic differential equations (SDEs) play a crucial role in modeling and analyzing various complex phenomena in finance, physics, and engineering. One key aspect of working with SDEs is parameter estimation, which involves estimating the unknown parameters of the equations based on observed data. In this article, we will delve into the intricacies of parameter estimation in SDEs, providing comprehensive lecture notes on the topic.
Understanding Stochastic Differential Equations
Before we dive into parameter estimation, let's first establish a solid understanding of stochastic differential equations. SDEs are mathematical equations that describe the evolution of a system in continuous time under the influence of random fluctuations. They are widely used to model systems that are subject to both deterministic dynamics and random noise.
An SDE is typically formulated as follows:
4.3 out of 5
Language | : | English |
File size | : | 4642 KB |
Screen Reader | : | Supported |
Print length | : | 268 pages |
dX(t) = f(X(t),θ)dt + g(X(t))dW(t)
Where X(t) represents the state variable at time t, f(X(t),θ) denotes the deterministic drift term, g(X(t)) represents the diffusion term, θ is the unknown parameter vector, dt is an infinitesimal time step, and dW(t) represents a Wiener process or Brownian motion which accounts for the random fluctuations. The goal of parameter estimation is to estimate θ based on observed data.
The Challenges of Parameter Estimation in SDEs
Parameter estimation in SDEs presents unique challenges compared to deterministic differential equations due to the presence of random noise. The primary difficulty lies in the fact that the likelihood function, which is commonly used for parameter estimation, is not readily available in closed-form for SDEs. This necessitates the use of numerical techniques to estimate the parameters.
The commonly employed approach for parameter estimation in SDEs is the maximum likelihood estimation (MLE) method, which involves maximizing the likelihood function with respect to the unknown parameters. However, directly maximizing the likelihood function is often infeasible due to its complexity.
As a result, specialized numerical algorithms, such as the Euler-Maruyama method, the Milstein scheme, or the stochastic gradient descent (SGD) algorithm, have been developed to approximate the likelihood function and optimize the parameter estimation.
Parameter Estimation Methods
There are several methods available for parameter estimation in SDEs, each with its unique advantages and limitations. Here, we discuss some of the frequently used methods:
1. Maximum Likelihood Estimation (MLE)
The MLE method aims to find the parameter values that maximize the likelihood function. It involves formulating the likelihood function based on the observed data and then optimizing it numerically using algorithms such as the Newton-Raphson method or the expectation-maximization (EM) algorithm.
2. Bayesian Estimation
Bayesian estimation combines prior knowledge about the parameters with the likelihood function to obtain the posterior distribution of the parameters. This approach allows for the incorporation of prior beliefs and the quantification of uncertainties in the parameter estimates.
3. Markov Chain Monte Carlo (MCMC)
MCMC methods, such as the Metropolis-Hastings algorithm or the Gibbs sampler, are widely used for parameter estimation in SDEs. These methods generate samples from the posterior distribution of the parameters, allowing for the estimation of the parameter values and their uncertainties.
4. Expectation-Maximization (EM) Algorithm
The EM algorithm is an iterative method used to estimate parameters when there are unobserved or latent variables. In the context of SDEs, the EM algorithm is often employed in conjunction with the Euler-Maruyama method to estimate the unknown parameters.
Applications of Parameter Estimation in SDEs
Parameter estimation in SDEs finds applications across various disciplines. Here are a few examples:
1. Finance
In finance, SDEs are widely used to model the dynamics of asset prices and interest rates. Parameter estimation in SDEs enables the calibration of financial models and the assessment of market risk.
2. Physics
Parameter estimation in SDEs plays a crucial role in modeling physical phenomena, such as the diffusion of particles in a medium or the movement of biological cells. It allows scientists to infer the underlying dynamics and better understand the system.
3. Engineering
Engineers often employ SDEs to model and analyze dynamic systems subject to noise, such as control systems or signal processing. Parameter estimation in SDEs enables engineers to optimize system performance and enhance robustness.
Parameter estimation in stochastic differential equations is a challenging yet essential task for understanding and modeling various complex phenomena. In this comprehensive guide, we have explored the intricacies of parameter estimation in SDEs, discussing the challenges, methods, and applications of this technique. By effectively estimating the unknown parameters, we can gain valuable insights into the dynamics of complex systems, enabling better decision-making and improved performance across various disciplines.
4.3 out of 5
Language | : | English |
File size | : | 4642 KB |
Screen Reader | : | Supported |
Print length | : | 268 pages |
Parameter estimation in stochastic differential equations and stochastic partial differential equations is the science, art and technology of modeling complex phenomena. The subject has attracted researchers from several areas of mathematics. This volume presents the estimation of the unknown parameters in the corresponding continuous models based on continuous and discrete observations and examines extensively maximum likelihood, minimum contrast and Bayesian methods.
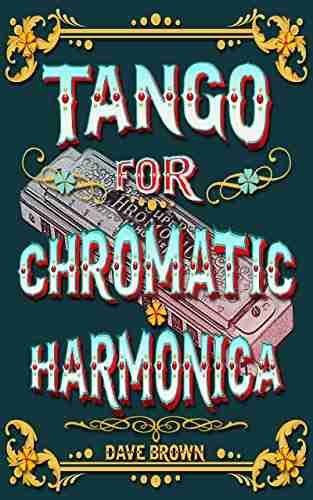

Tango For Chromatic Harmonica Dave Brown: Unleashing the...
The hauntingly beautiful sound of the...
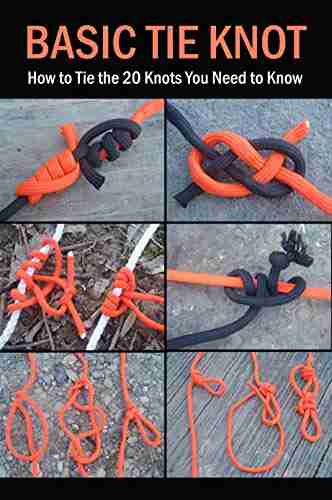

How To Tie The 20 Knots You Need To Know
Knot-tying is an essential...
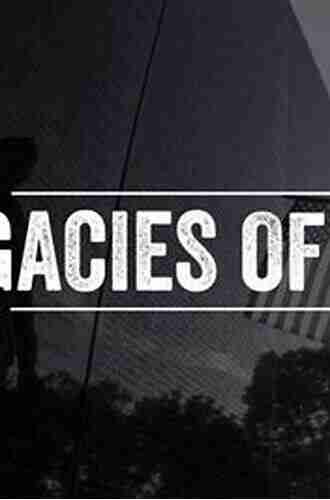

The Politics Experiences and Legacies of War in the US,...
War has always had a profound impact...
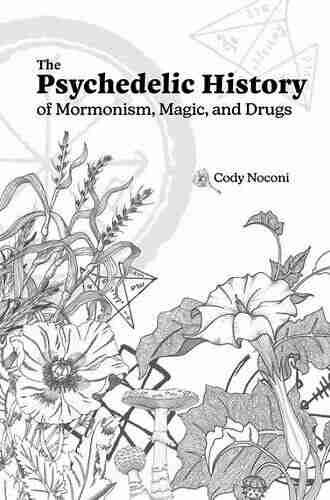

The Psychedelic History Of Mormonism Magic And Drugs
Throughout history, the connections between...
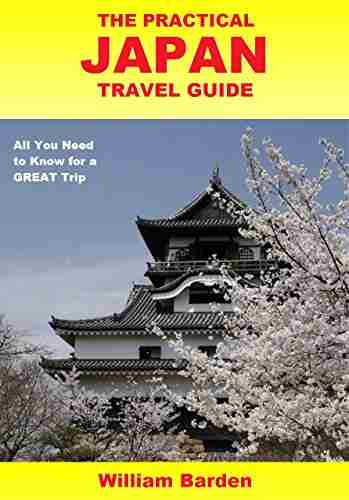

The Practical Japan Travel Guide: All You Need To Know...
Japan, known for its unique...
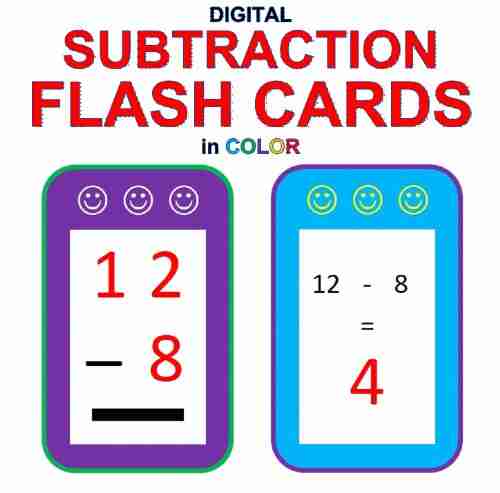

Digital Subtraction Flash Cards in Color: Shuffled Twice...
Mathematics is an essential...
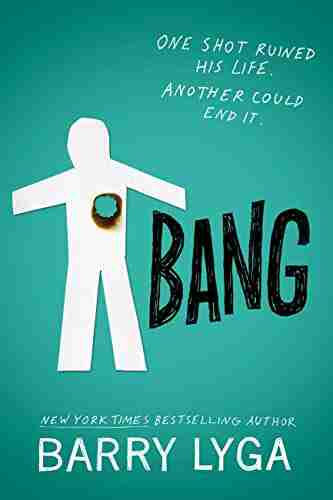

Unveiling the Enigma: Explore the Fascinating World of...
Hello, dear readers! Today, we have a...
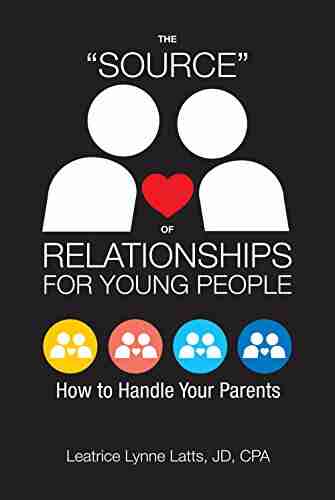

How To Handle Your Parents - A Comprehensive Guide
Are you having trouble dealing with your...
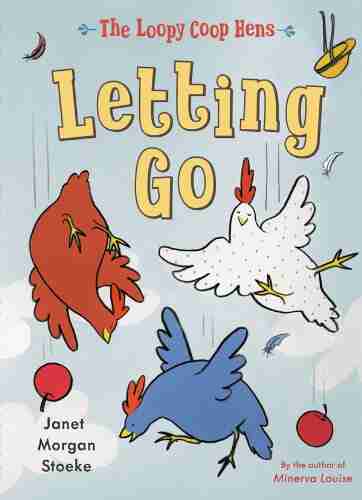

The Loopy Coop Hens Letting Go: A Tale of Friendship and...
Once upon a time, in a peaceful...
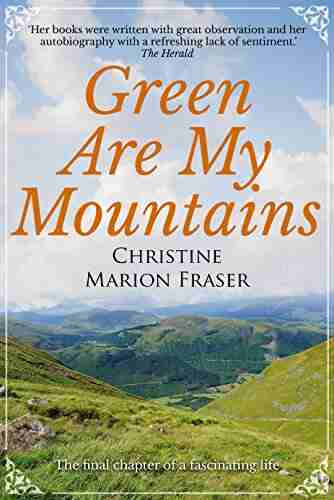

Green Are My Mountains: An Autobiography That Will Leave...
Are you ready to embark on an...
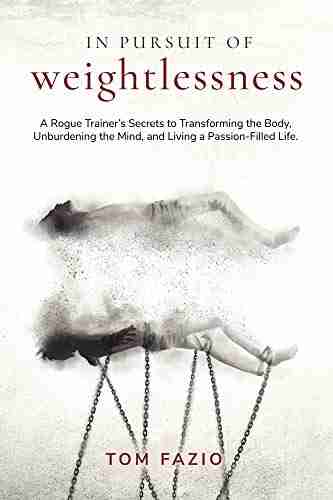

Rogue Trainer Secrets To Transforming The Body...
In this fast-paced...
Light bulbAdvertise smarter! Our strategic ad space ensures maximum exposure. Reserve your spot today!
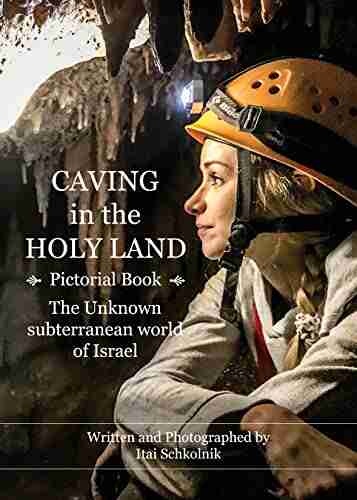

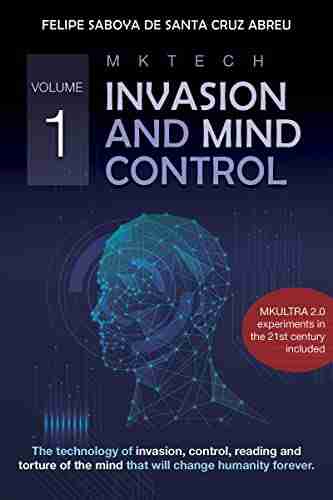

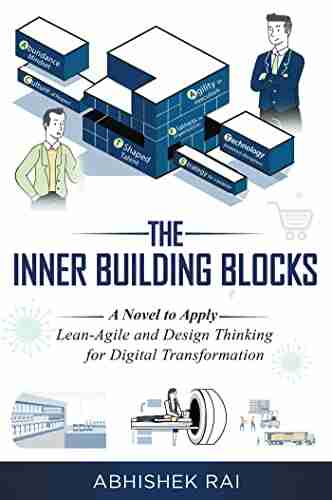

- Fred FosterFollow ·15.3k
- Nathaniel HawthorneFollow ·18.8k
- Quincy WardFollow ·10.4k
- Clay PowellFollow ·4.2k
- Fabian MitchellFollow ·8.7k
- Lee SimmonsFollow ·7.4k
- Mark MitchellFollow ·7.7k
- Paul ReedFollow ·14.1k